Share
The Department for Work and Pensions (DWP) has announced a pre-tender opportunity under its Nexus AI program, inviting suppliers to provide AI services across various capabilities. While this marks a step forward in the government’s adoption of AI, the approach raises significant concerns. Instead of concentrating on well-defined, citizen-focused outcomes, this procurement is technology searching for a problem.
AI should be used to address real-world challenges, not as an end in itself. The government’s approach to AI procurement must shift away from generic, technology-first initiatives and instead prioritise measurable outcomes that improve public services. Otherwise, AI risks becoming a costly experiment rather than a force for transformation.
The Issue with AI-First Procurement
The DWP’s Prior Information Notice (PIN) outlines ambitious goals, including proof-of-concept AI experiments, the implementation and support of AI systems, risk management, governance, engagement, and the development of common components. While these objectives sound promising, what is notably absent is a clear identification of the specific problems that AI is intended to address.
When procuring technology in the public sector, it is crucial to understand users’ needs, identify inefficiencies, and define the desired outcomes. Failing to connect directly with citizens’ needs can lead to misallocating resources on projects that may fail to achieve meaningful impact.
Lessons from Experience
At Modular Data, we’ve seen first-hand how an outcomes-driven approach delivers value. Our work in government has always focused on using data as a product to solve specific business challenges. Instead of deploying AI for its own sake, we’ve built tools that address inefficiencies in data management, accessibility, governance, and decision-making, ensuring that AI and automation are applied where they enhance operations.
For example, decision support systems can help operational staff assess risk more accurately, but the foundation of this capability is high-quality, well-governed data. Delving this business value may not require AI at all. The first stage might make information available to those who need it securely. Deploying AI without solving data quality issues first would be ineffective. This is precisely the kind of oversight that can occur when AI is procured without an outcome-driven strategy.
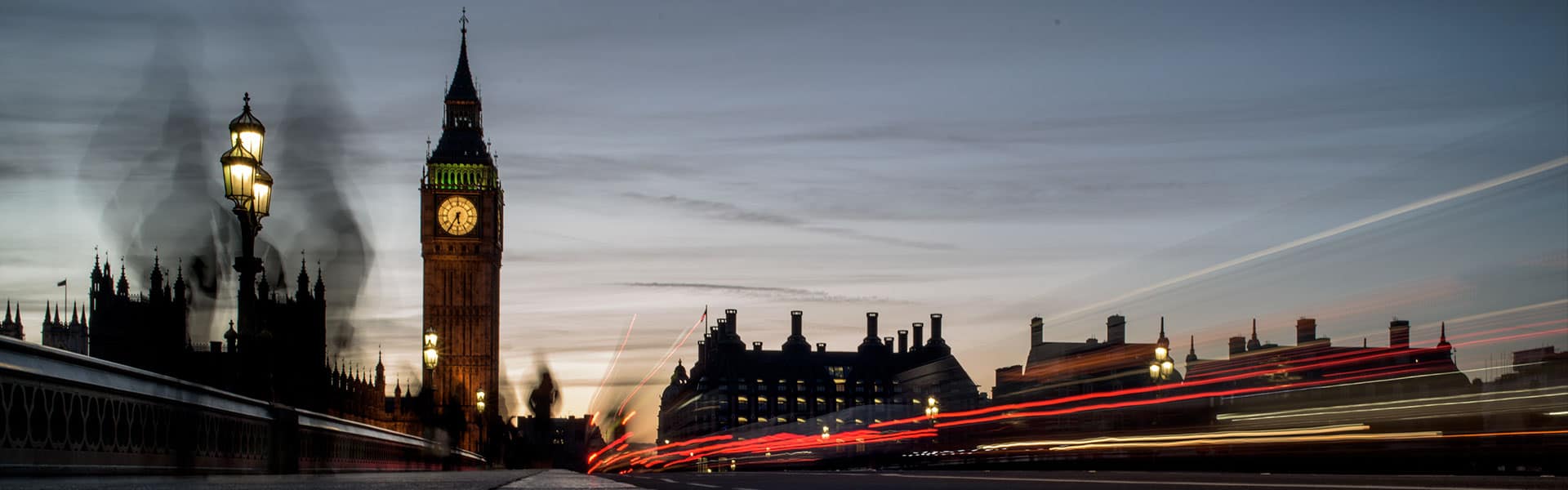
Outcomes, not technology, should lead to AI
With AI’s increasingly central role in government digital transformation, public funds must be spent to maximise societal benefit. AI must be framed as an enabler of change, not the driving force.
DWP’s approach should begin with well-defined challenges:
- How can data reduce waiting times for benefits processing?
- How can it help identify fraud while ensuring fairness?
- How can predictive analytics improve employment support services?
AI can be deployed strategically and effectively by starting with these real-world questions. Unfortunately, the procurement language lacks this clarity and presents AI as a standalone initiative, disconnected from genuine service transformation.
The Risk of Repeating Past Mistakes
We have encountered challenges similar to those experienced by previous government AI initiatives. The UK government has appropriately highlighted the importance of responsible AI in its AI Playbook; however, executing these principles has been inconsistent. One significant risk of an AI-first approach is that it can result in costly pilot programs and proof-of-concept projects that fail to transition into meaningful implementation. Government AI initiatives often find it challenging to create a positive impact without a clear roadmap that outlines the journey from experimentation to service-wide deployment.
We only need to look at past examples of failed technology procurement in government to see the risks. Expensive, centralised IT systems meant to modernise public services have frequently fallen short due to a lack of alignment with user needs. AI risks falling into the same pattern—high upfront investment with little tangible return if procured incorrectly.
A Call for Better AI Procurement Strategies
A different procurement approach is needed if DWP wants to harness AI for public benefit. Here’s what we recommend:
1. Define the Outcomes First: Procurement should start by articulating AI’s problems clearly and ensuring that solutions are outcome-driven.
2. Focus on Data Readiness: AI is only as effective as the data that powers it. Investing in high-quality, well-governed data should be a prerequisite.
3. Prioritise Explainability and Trust: Any AI system used in public services should be transparent, explainable, and aligned with ethical AI principles.
4. Ensure Long-Term Viability: AI should not be confined to short-term proof-of-concepts. There must be a roadmap from pilot projects to full-scale deployment.
5. Embrace a Modular Approach: Rather than relying on large, centralised AI solutions, we should prioritise modular, interoperable components. This promotes adaptability and minimises the risk of vendor lock-in.
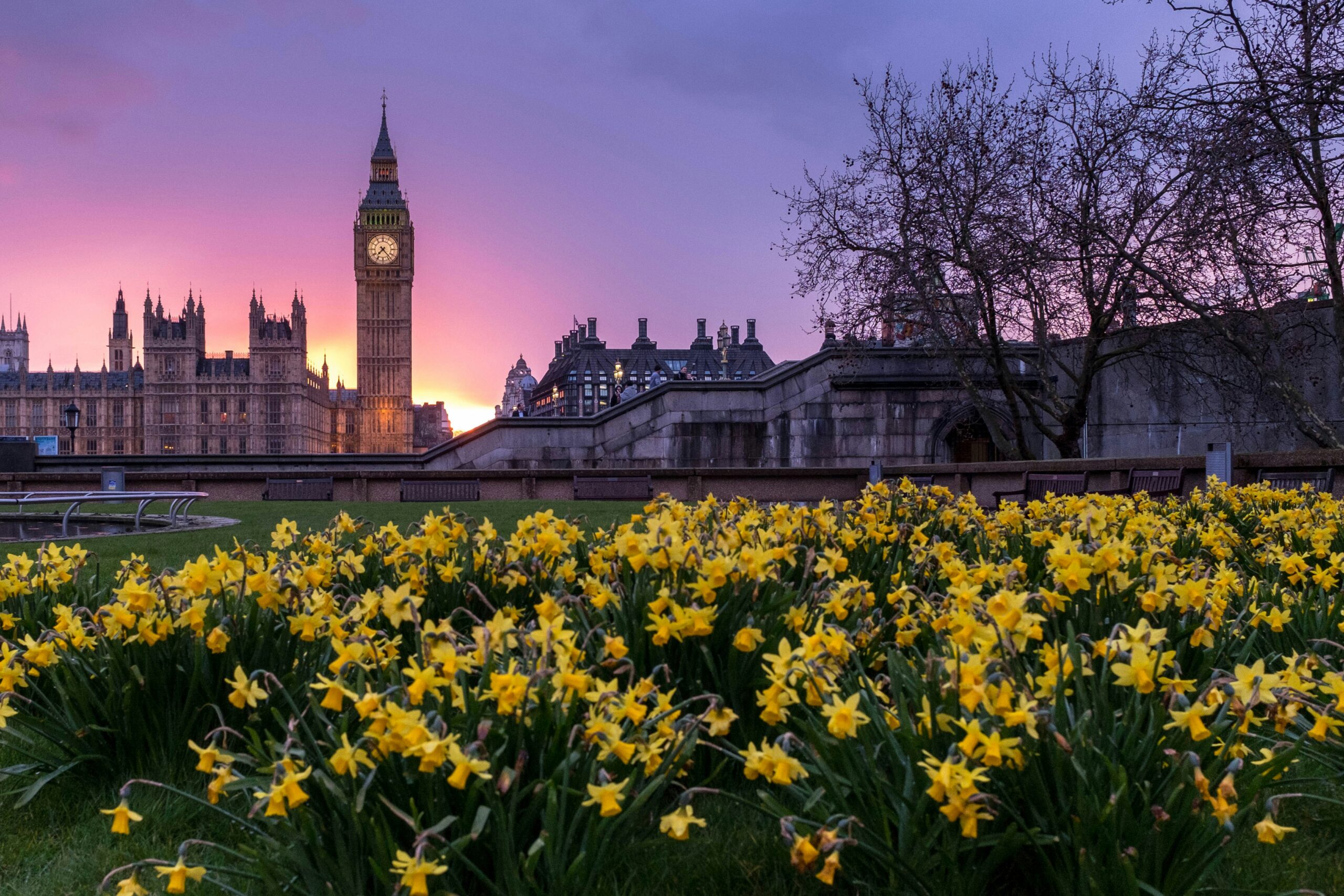
Conclusion
The DWP’s AI procurement plans underscore a broader challenge in the government’s technology strategy. While AI is a powerful tool, it can become a costly distraction without an explicit focus on outcomes. Instead of taking a solution-first approach, government agencies should consider AI in relation to real-world challenges and citizens’ needs.
At Modular Data, we are committed to helping the public sector unlock the value of AI responsibly and effectively. Outcomes, not hype, should lead AI, and procurement should be shaped by tangible public benefit rather than abstract technological ambition.
If AI is to transform public services, it must be built around the citizens who rely on them, not simply deployed for perceived innovation.