Why open-source AI and data ethics are the future of public services
The recent Meta hackathon on AI in the public sector shows that the trend towards integrating AI into public services has put us in a pivotal moment of government innovation. It’s exciting to be in the industry right now experiencing how, more and more, we’re beginning to explore the many ways AI can benefit the public sector.
However, responsible AI adoption requires balancing ambition for technological progress with ethical accountability, especially when using closed-source, proprietary AI platforms in the public domain. I’ve always been an advocate of open-source tools and platforms. They foster a culture of transparency, openness, and ultimately, trust. It’s no different when it comes to AI.
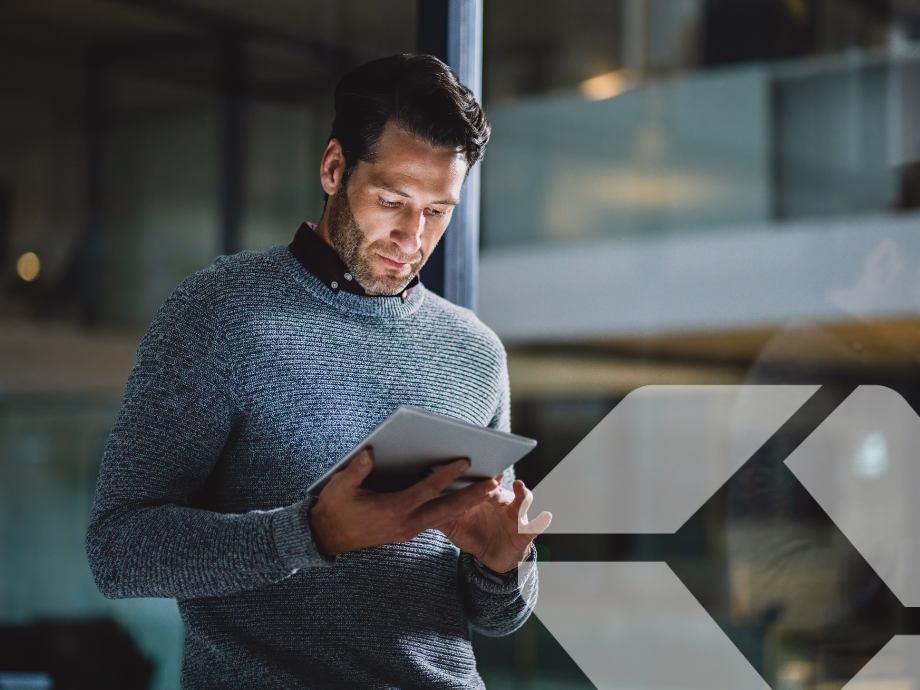
Open-source AI enforces public accountability
Closed-source AI platforms can be powerful but pose significant challenges around transparency and accountability. Their proprietary design creates a “black box” effect, hiding how decisions are made and limiting public oversight. Additionally, the vendor lock-in risks can hinder innovation and flexibility, and tie public institutions to expensive long-term contracts.
In contrast, open-source AI platforms provide more transparency and flexibility. They promote collaboration and allows governments, researchers, and the public to scrutinise and improve systems. This approach builds trust, drives innovation, and keeps AI focused on public needs instead of corporate goals.
Ethics as the cornerstone of public sector AI
Any application of AI in public services must follow strong ethical guidelines, such as those outlined in UNESCO’s Recommendation on the Ethics of Artificial Intelligence. Key priorities include:
- Transparency: AI systems should be understandable, ensuring decisions are clear and accountable, to foster public trust.
- Human Oversight: Humans must remain responsible for decisions and avoid an over-reliance on AI.
- Fairness and Inclusivity: AI should be designed to avoid, or at least mitigate, bias, especially in critical areas like healthcare, welfare, and justice.
- Sustainability: Given the often-high energy demands of AI, effort should be taken to prioritise sustainability, minimise energy use, and align with environmental goals.
Critically from an ethical point of view, the UNESCO framework bans AI-driven mass surveillance and social scoring, stressing that AI must protect civil liberties and privacy.
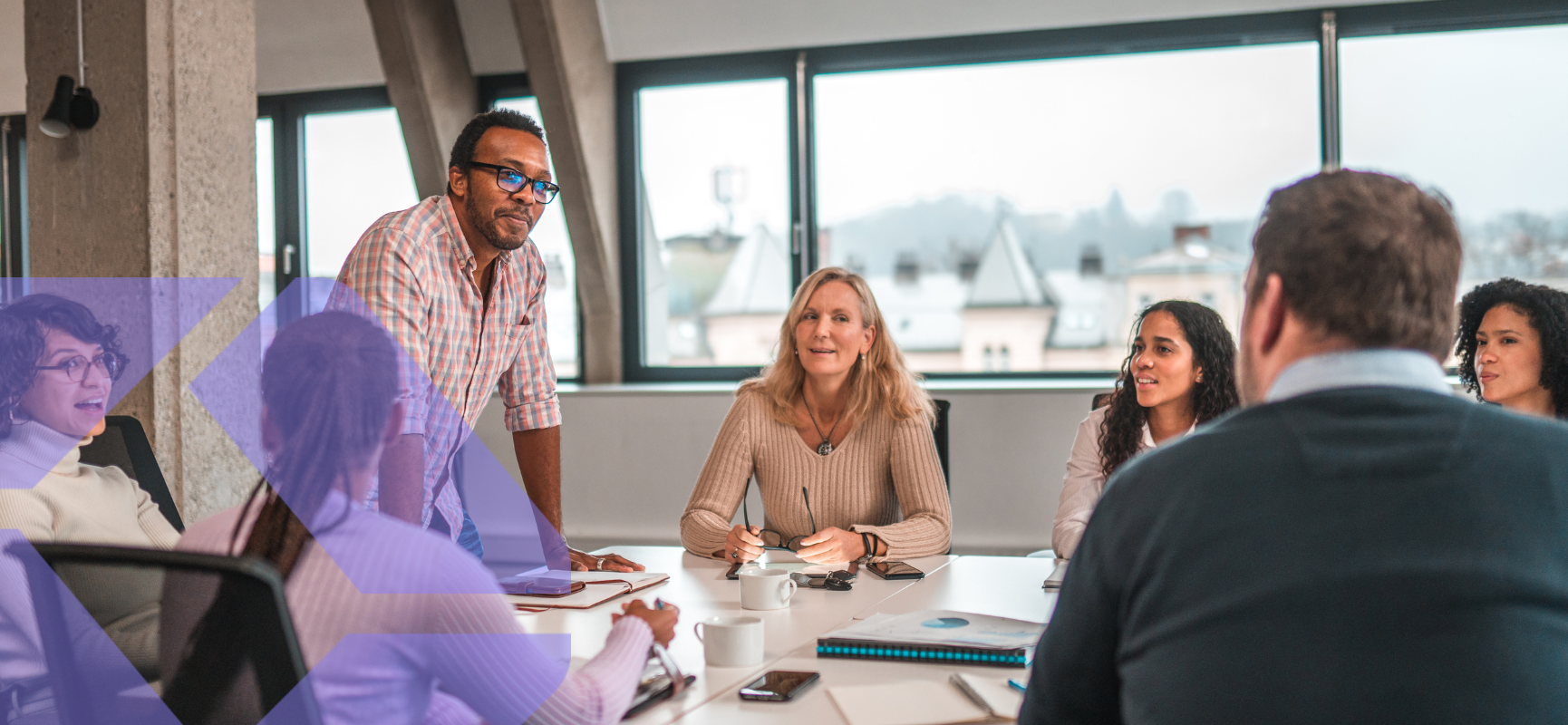
A roadmap for public sector AI
Integrating AI into public services requires a clear data maturity roadmap. Our approach at Modular Data’s shows the value of moving from basic data management to advanced cloud systems for real-time, actionable insights. This ensures AI development is both effective and fair.
Key steps to building a more mature data roadmap include:
- Building strong data governance frameworks to ensure quality, security, and compliance.
- Promoting AI literacy in public institutions to empower decision-makers and build trust in AI initiatives.
- Conducting ethical impact assessments to ensure AI serves public interests.
Collaboration for sustainable AI innovation
The future of AI in public services depends on collaboration among governments, private companies, and civil society to ensure systems are both effective and ethical. This supports innovation and growth whilst still safeguarding public values.
The choice for public-sector organisations exploring AI adoption is clear: choose transparency, follow ethical principles, and adopt open, flexible solutions. This approach means we can unlock AI’s potential while prioritising the public good.
Looking to innovate with AI? Learn more about how we can create transparent, ethical AI models that deliver real-time value.
Not quite ready to take the leap? Explore our range of AI workshops to understand more about how your organisation could benefit from AI.